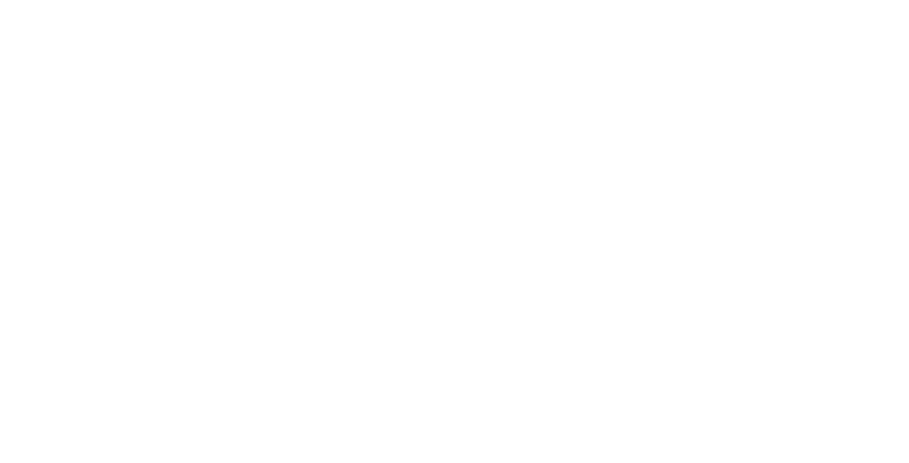
7
Genetic Resources. We also use these groups to evaluate a range of terms proposed to replace DSI,
as shown in Table 4, which is a key reference for the reader to understand the different groups
proposed to evaluate the concept of DSI in this study. It is evident from these evaluations that
terminology is readily available to describe DSI with narrow subject matter as proposed in Group 1.
These terms include Genetic Resource Sequence Data (GRSD); Genetic Sequences (GS); Genetic
Sequence Data/Information (GSD/GSI); and Nucleotide Sequence Data (NSD)). Consequently, the
terms Digital Sequence Data (DSD), Genetic Resource Sequence Data (GRSD) or Genetic Resource
Sequence Data and Information (GRSDI), could be used to describe subject matter of intermediate
scope as proposed in Group 2, depending on the interpretation adopted. None of the terms
proposed to date appear to adequately capture an intermediate range comprising information
associated with the additional biosynthesis of a genetic resource as proposed by Group 3. Finally,
terminology is also readily available to describe subject matter with broad scope as proposed in
Group 4. Overall, the four logical groups proposed in this study provide a nuanced alternative to the
2018 AHTEG list and so may better assist in clarifying the concept and scope of DSI, however,
appropriate terminology will need to be evaluated, particularly for the intermediate groups.
The proximity of information to the underlying genetic resource determines whether it is possible to
accurately identify or infer the source from which it is derived. This is possible to differing degrees in
the case of RNA and protein sequences, however, it becomes much more challenging with
biosynthetic information and impossible with subsidiary information. Accordingly, the proximity of
data/information has significant implications for traceability to a particular genetic resource and also
in identifying the source of information, including whether it has been generated through the
utilization of a genetic resource or independently. In a system in which the traceability of DSI is
important, a narrow scope of DSI subject matter appears better suited given the technical difficulties
in identifying or inferring origin, whereas if traceability is not important a broader scope of subject
matter may be able to be accommodated.
The study identified several key issues, as well as potential solutions which should be considered and
resolved as a priority in order to help clarify the concept of DSI. The first two questions are: 1.) how
far along the flow from genetic resource onwards to DNA, RNA, protein sequences and metabolites
‘DSI’ can be considered to extend; and 2.) whether DSI includes both data and information and the
extent to which data has been processed before it can be considered information. Both questions
can be resolved by adopting one of the four clearly defined proposed groups to clarify the scope of
DSI subject matter. The third question is whether certain sequence information should be excluded
from the scope of DSI subject matter, including sequences below a certain length, non-coding DNA,
epigenetic heritable factors and modified DNA/RNA/proteins. A sequence below 30 nucleotides may
not be unique, and this may provide a lower cut-off for sequence length. Non-coding DNA,
epigenetic heritable factors and DNA/RNA/proteins modified naturally all have functions suggesting
it might be logical to consider them for inclusion in the DSI subject matter. Conversely synthetically
modified DNA, RNA or proteins cannot be said to have a natural functional role and so on this basis
could be considered not to be an inherent part of the underlying genetic resource. Irrespective of
whether the logical groups proposed in this study are adopted, it is anticipated that the priority
issues identified in this study and a greater appreciation of the extent to which DSI across a range of
sectors in the life sciences, will assist the deliberations and the recommendations by the new Ad Hoc
Technical Expert Group (AHTEG) on Digital Sequence Information on Genetic Resources which will
consider the studies commissioned pursuant to decision 14/20 at the 14
th
Conference of the Parties
to the Convention on Biological Diversity.