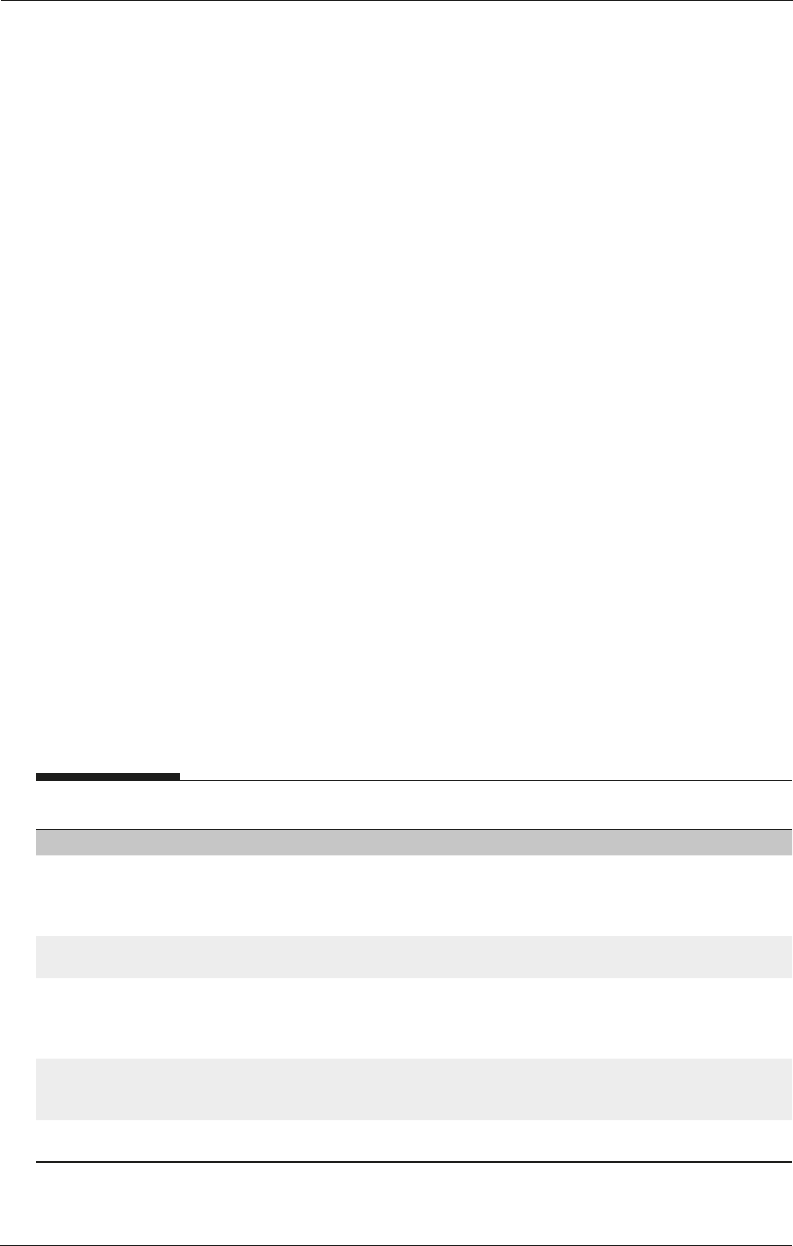
An, Jakabovics, Liu, Orlando, Rodnyansky, Voith, Zielenbach, and Bostic
316
need to generate profits for their shareholders. In contrast, nonprofit developers tend to focus more
on neighborhood improvements and affordable housing provision. Nonprofits, consequently, are
more likely to develop properties in poorer areas. Their properties frequently target lower-income
households, and they also are more likely to target people with disabilities, the homeless, seniors,
and other “special” populations (Johnson, 2012; Silverman and Patterson, 2011). Because they
serve more disadvantaged populations, nonprofit properties often charge lower rents. Bratt and
Lew (2016), for instance, found that nonprofit-sponsored LIHTC developments had a higher
proportion of units with a low rent-fair market rent ratio than for-profit developments.
Overall, the cost of developing a LIHTC project tends to be higher for a nonprofit developer than
for its for-profit counterparts. Some of that difference can be attributed to nonprofits being more
likely to engage in the rehabilitation of existing properties and offering more services to tenants.
Whereas for-profit developers are more likely to engage in new construction, with models that
can be replicated across sites, nonprofits often need to develop project-specific designs for existing
properties (Silverman and Patterson, 2011). At least in metropolitan Richmond, Virginia, nonprofit
developers of LIHTC properties have been more likely to incorporate rehabilitation, certified
property management, and standard-use terms in their properties than their for-profit counterparts
(Johnson, 2012). These factors can contribute to greater operating costs. Many nonprofit
developers also struggle to obtain capital from conventional lenders and, therefore, are forced to
piece together different subsidies, particularly if they try to serve very low-income households. This
process can take time and ultimately drive up overall project costs.
Development costs notwithstanding, some evidence exists that nonprofit-developed affordable
housing complexes have at least similar, and potentially more positive, effects on surrounding
home values than properties developed by for-profit firms. Exhibit 4 summarizes the relatively
sparse literature on the issue.
Exhibit 4
Selected Analyses of Spillover Price Effects from Nonprot and For-Prot Affordable
Housing Developments
Study Market Key Developer-Related Findings
Goetz, Lam,
and Heitlinger
(1996)
Minneapolis, MN Subsidized multifamily properties developed by nonprot CDCs
enhanced the value of surrounding market-rate homes by 86 cents per
square foot. Publicly subsidized housing owned by private for-prots had
a negative 82 cent per square-foot effect on surrounding home prices.
Smith (2003) Indianapolis, IN For 13 years, home prices in areas with signicant CDC activity increased
7.14% relative to homes in non-CDC neighborhoods.
Ellen and
Voicu (2007)
New York City, NY Nonprot-developed, smaller affordable housing properties had larger
home price spillover effects than similar for-prot developed properties.
The price-value effects associated with nonprot-developed projects
were more stable over time.
Deng (2011a) Santa Clara
County, CA
LIHTC projects developed by HPN-member* nonprots had 4 to 6
percentage points higher effects on surrounding values than properties
developed by for-prots or by non-HPN member nonprots.
Edmiston
(2018)
Kansas City, MO CDC investments in owner-occupied, single-family homes contributed to an
11.8% increase in home prices within 500 feet of the targeted properties.
CDC = Community Development Corporation. HPN = Housing Partnership Network. LIHTC = low-income housing tax credit.
* HPN is a national network of high-capacity nonprofit developers.